Solvers¶
The following classes provide different solvers for specific tasks.
Electric Potential¶
-
class
magnumfe.
ElectricPotential
(magnetic_region='magnetic', conducting_region='conducting', outermagnet_region='outermagnet', solver='cg', prec='default')¶ Computes the electric potential and current according to Ohm’s law. Boundray conditions can be given either as potential (Dirichlet) or current outflow (Neumann).
- Example
# Initialize state with magnetization state = State(...) # set a constant current inflow on contact1 facet-region state.j_boundary = {'contact1': Constant(1e12)} # set a constant potential on contact2 facet-region state.u_boundary = {'contact2': Constant(0.0)} # Create solver and hook into state via virtual attributes potential = ElectricPotential() state.u = potential.u() state.j = potential.j()
- Arguments
- conducting_region (
str
) - Name of the conducting region, defaults to “conducting”
- solver (
str
) - Solver for linear system (“cg”, “gmres”,...), defaults to “bicgstab”
- prec (
str
) - Preconditioner for linear system (“ilu”, “amg”, ...), default to “ilu”
- conducting_region (
- Required fields
- u_boundary
- Potential boundary conditions
- j_boundary
- Current boundary conditions
- Required material parameters
- sigma
- Electric conductivity
- C0
- Alternative to conductivity, see
SpinAccumulationForPotential
(\(C_0 = \sigma/2\))
Maxwell Solvers¶
Different methods are available for the solution of the magnetostatic Maxwell equations:
The open-boundary problem is handled by the direct Johnson-Nedelec FEM-BEM coupling method, which avoids the discretization of the other domain. The following jump conditions need to be considered at the surface of the magnetic material:
The magnetic flux density \(\vec{B}\) and the magnetic field \(\vec{H}\) are connected via a (nonlinear) material law. For the numerical solutioin with a Newton-like method, the material law is linearized around the actual bias point:
At the moment only \(H\)-based methods are implemented. The source of these methods is an external field \(H_\text{ext}\) (sometimes called impressed current vector potential). Any FieldTerm class can be used to create the source field for a Maxwell problem. If for example a known current density should act as sources of the magnetic field the OerstedField class can be used to calculate \(H_\text{ext}\).
MaxwellReduced
¶
Since the external field \(H_\text{ext}\) contains all rotational contibutions to the total field which are created by some current distribution, the remaining induced field \(H_\text{d}\) is irrotational and can therefor be described by a gradient field:
The reduced scalar potential \(u\) can be defined globally and always exists. Unfortunately cancelation effects can occur if magnetic materials with high susceptibility are considered. In this case the induced field and the external field nearly cancel each other, which may lead to large numerical errors. Nevertheless the reduced scalar potential is often useful due to its simplicity.
Using the direct Johnson-Nedelec FEM-BEM coupling method leads to the following discretized system of equations:
From the mathematical point of view using piecewise linear and continuous basis functions \(\Lambda_i\) for the potential and piecewise constant basis functions \(\mathbb{1}_n\) for the normal derivative seems to be the natural choice for a lowest order discritization. Nevertheless the Johnson-Nedelec coupling should be stable for arbitrary combinations of function spaces.
-
class
magnumfe.
MaxwellReduced
(lump=True, region='maxwell', tol=1e-16, maxiter=20)¶ This class solves the magnetostatic Maxwell equations using a reduced scalar potential formulation as introduced in [Bruckner2012].
- Example
mesh = UnitCubeMesh(10,10,10) state = State(mesh, scale = 1e-9) state.maxwell_material = MaxwellMaterial.Linear(chi=1e3) state.Hext = ExternalField(Constant((0.0, 0.0, 1.0))).h(state) solver = MaxwellReduced() Hd = solver.Hd(state) Htot = solver.Htot(state) M = solver.M(state) B = solver.B(state)
Note
The external field is assumed to be in state.Hext.
Note
This class requires the PETSc Backend to be enabled.
- Arguments
- lump (
bool
) - Flag, if set to
True
external field terms are computed with mass lumping - prec_solver (
string
) - Solver for preconditioning system, either ‘LU’ or ‘GMRES’, defaults to ‘LU’
- region (
string
) - The region where the Maxwell equations are solved, defaults to ‘maxwell’
- tol (
float
) - Threshold for the tolerance within the Newton iteration
- maxiter (
int
) - Maximum number of Newton iterations
- lump (
MaxwellTotal
¶
An alternative scalar potential formulation, which does not suffer from numerical cancelation errors, is the total scalar potential. In contrast to the reduced scalar potential, the total scalar potential directly describes the total field:
Since this formulation is only allowed in regions without current density, it is necessary to devide the global problem into inner and other region. While the total scalar potential can only be defined within the inner region, a reduced scalar potential is used to describe the outer region (where some currents are needed to create the source field \(H_\text{ext}\). Additionally the total scalar potential is only well defined if the inner region is singly-connected (artificial cuts can be used to create a singly-connected domain). Furthermore since the potential description changes from inner to outer region the formulatioin of the jump conditions at the boundary of the magnetic domain, requiers the knowledge of a potential of the external field \(u_\text{ext}\). Putting everything together the following equations can be derived for the total scalar potential:
Using the direct Johnson-Nedelec FEM-BEM coupling method leads to the following discretized system of equations:
-
class
magnumfe.
MaxwellTotal
(lump=True, region='maxwell')¶ This class solves the magnetostatic Maxwell equations using a total scalar potential formulation as introduced in [Bruckner2012].
- Example
mesh = UnitCubeMesh(10,10,10) state = State(mesh, scale = 1e-9) state.maxwell_material = MaxwellMaterial.Linear(chi=1e3) state.Hext = ExternalField(Constant((0.0, 0.0, 1.0))).h(state) solver = MaxwellTotal() Hd = solver.Hd(state) Htot = solver.Htot(state) M = solver.M(state) B = solver.B(state)
Note
The external field is assumed to be in state.Hext.
Note
This class requires the PETSc Backend to be enabled.
- Arguments
- lump (
bool
) - Flag, if set to
True
external field terms are computed with mass lumping - prec_solver (
string
) - Solver for preconditioning system, either ‘LU’ or ‘GMRES’, defaults to ‘LU’
- region (
string
) - The region where the Maxwell equations are solved, defaults to ‘maxwell’
- lump (
StringSolver
¶
magnum.fe implements the string method as introduced in [E2007] for the computation of energy barriers between two defined magnetic states that usually mark local minimia in the energy landscape. The basic working principle of the string method is illustrated in Fig. 19.
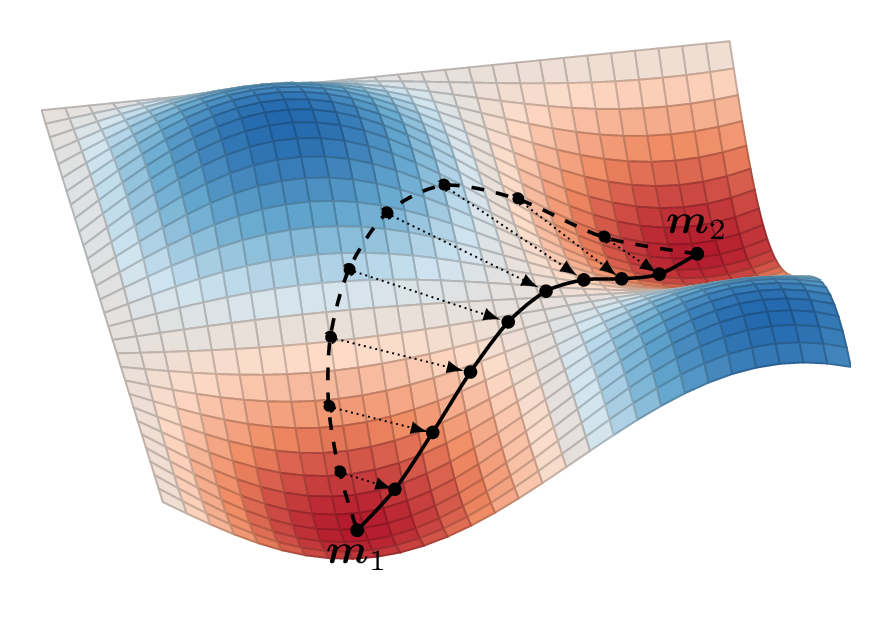
Fig. 19 Visualization of the string method used to find the energy barrier between two energy minima. The dashed line is the start path discretized by 10 images shown as dots. The solid line is the resulting minimum energy path as computed by the string method.
The starting point for the energy barrier minimization is an arbitrary path between the magnetization configurations \(\vec{m}_1\) and \(\vec{m}_2\) discretized by a number of transitional magnetization states referred to as images. The minimum energy barrier between \(\vec{m}_1\) and \(\vec{m}_2\) is then computed by iteratively relaxing the initial transition path. Each step of this procedure consists of two operations. In the first operation every image is driven towards an energy minimum by a steepest descend method. In a second operation the images on the resulting path are rearanged in an equidistant fashion by a spline interpolation as suggested in [E2007]. When converged the whole information of the minumum energy path, including both images and energies, is made available to the user.
-
class
magnumfe.
StringSolver
(state, terms=[], num_images=20, fix_start=False, fix_end=False, region='magnetic')¶ This class implements the string method for energy barrier calculations as introduced in [E2007]. The images are stored in a
MagnetizationCollection
object that acts as a list ofdolfin.Function
objects and moreover provides convenience wrappers to access the energies and averaged magnetization for the individual images.- Example
string = StringSolver([ExchangeField(), UniaxialAnisotropyField()], 20) # create initial images images = string.level([ Constant((0,0,-1)), Constant((0,1,0)), Constant((0,0,1)) ]) # perform 10 string steps for i in range(10): string.step(images) # print energy barrier print max(images.E) - min(images.E) # print average magnetization of first image print images[0].m # save all images f = File("m.pvd") for image in images: f << image
- Arguments
- terms ([
LLGTerm
]) - list of LLG contributions to be considered for energy minimization
- num_images (
int
) - number of images used for minimization
- fix_start (
bool
) - fix start image
- fix_end (
bool
) - fix end image
- region (
str
) - region on which the LLG is solved for minimization
- terms ([
-
level
(images)¶ Rearanges images in an equidistant manner by spline interpolation and returns the result as
MagnetizationCollection
object as required by thestep
method. While the number of input images is arbitrary, the number of ouput images is defined by thenum_images
attribute of the class.- Arguments
- images (
list
/MagnetizationCollection
) - images to be leveled provided either as Python
list
orMagnetizationCollection
- images (
- Returns
MagnetizationCollection
- the leveled images
-
step
(images, h=1e-11)¶ Performs a single string method step. The images are provided as
MagnetizationCollection
object as provided by thelevel
method and are update inplace. The result of the step is already leveled.- Arguments
- images (
MagnetizationCollection
) - the magnetization images
- h (
float
) - size of the steepest descend step used to relax the images
- images (
Minimizer_BB
¶
-
class
magnumfe.
Minimizer_BB
(terms=[], region='magnetic', tau_min=1e-10, tau_max=1e-05, dm_max=10000.0, samples=10)¶ This class implements the direct energy minimizing algorithm introduced in [Exl2014].
Note
This feature is experimental.
- Example
state = State(mesh, m = Constant((1.0, 0.0, 0.0)) minimizer = Minimizer_BB([ExchangeField()]) minimizer.minimize(state)
- Arguments
- terms ([
LLGTerm
]) - List of LLG contributions to be considered for energy minimization
- region (
str
) - region on which the energy is minimized
- tau_min (
float
) - minimum step size
- tau_max (
float
) - maximum step size
- dm_max (
float
) - stop criterion given as supremum norm of dm/dt
- sample (
int
) - number of subsequent steps the stop criterion has to be fulfilled
- terms ([
Minimizer_LBFGS
¶
-
class
magnumfe.
Minimizer_LBFGS
(terms=[], region='magnetic', **args)¶ This class implements the direct energy minimizing algorithm based on L-BFGS iteration. For details see [Schrefl???]_.
Note
This feature is experimental.
- Example
state = State(mesh, m = Constant((1.0, 0.0, 0.0)) minimizer = Minimizer_LBFGS([ExchangeField()]) minimizer.minimize(state)
- Arguments
- terms ([
LLGTerm
]) - List of LLG contributions to be considered for energy minimization
- region (
str
) - region on which the energy is minimized
- maxiter (
int
) - maximum number of BFGS iterations (default = 200)
- tol (
float
) - Tolerance to be reached (default = 1e-6)
- m (
str
) - rank of the hessian approximation (default = 5)
- terms ([
[Bruckner2012] | (1, 2) 3D FEM–BEM-coupling method to solve magnetostatic Maxwell equations. Journal of Magnetism and Magnetic Materials, 324(10), 1862-1866 |
[Exl2014] | Exl, L., Bance, S., Reichel, F., Schrefl, T., Stimming, H. P., & Mauser, N. J. (2014). LaBonte’s method revisited: An effective steepest descent method for micromagnetic energy minimization. Journal of Applied Physics, 115(17), 17D118. |
[E2007] | (1, 2, 3) Weinan, E., Ren, W., & Vanden-Eijnden, E. (2007). Simplified and improved string method for computing the minimum energy paths in barrier-crossing events. The Journal of Chemical Physics, 126(16), 164103. |